MACHINE LEARNING
Discovering physics laws from observation data
Various phenomena in natural and engineering systems, including the pendulum, planetary orbits, fluid dynamics, and lasers, can be regarded as dynamical systems, which are expressed by deterministic equations of motion. Interestingly, even when a system exhibits chaotic or irregular dynamics, its underlying equations are simple. However, it is generally difficult to discover underlying dynamical equations from experimental observations, particularly from high-dimensional observation datasets. In addition, it is more difficult to discover them if there is no prerequisite knowledge. The aim of this study is to discover hidden physics laws from experimental observation data using machine-learning techniques, including symbolic regression. By using these techniques, it is possible to extract (low-dimensional) reduced equations of motion from high-dimensional observables.
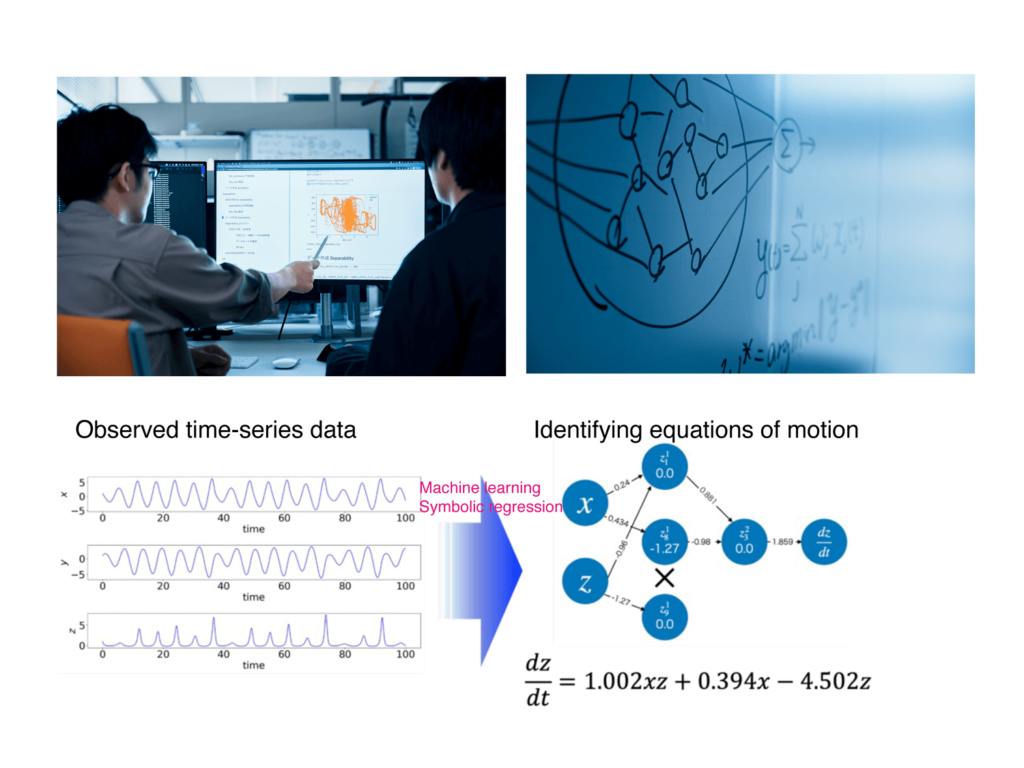